Rdatao; How often does data use change? – These days we live in world where information is the most common resource and it feeds every single second of our lives with its heavy-growing flow. The amount of data available, whether it be from e-commerce transactions or social platforms is mind-blowing. But real value comes from decoding this data of what actually is important. That is when data analysis comes to rescue. In today’s world, the tactic of utilizing significant data for practical consciousness requires no introduction – irrespective of whether or not it is in business otherwise tutorial inquiry and development. Whether you are a long time purveyor of the joy that is data analysis through R or just jumping into it for fun, we sure wish to see more off.
Delving Deeper: The Essence of Diagnostic Analysis
In the realm of data analytics, there are different techniques that help in deriving valuable information from unprocessed or raw data. Descriptive analysis Describe the dataset in a short manner, talk about all aspects of variable (variable could be any feature) This helps to unveil the patterns and trends within data.
Diagnostic analysis, on the flip side currently explores to a greater extent precisely what causes anything or even links between issues returning data which could certainly not happen relevance with signifies. It goes on to clarify the origins associated with specific occurrences or phenomena.
Predictive analysis uses advanced statistical algorithms and machine learning models to make future predictions by data that we have in our records. The ability to predict helps decision-makers foresee what will happens, plan and act in advance.
To go a level deeper, prescriptive analysis not only predicts but advises what actions should be taken to achieve desired results. It supports the procedure of making informed decisions by building on predictions made and analyzing data empowered from predictive models.
These different data analysis strategies address a variety of organizational requirements, helping firms utilize their resources in the best way possible to report winning operations benefits across offerings.
Mechanics of rdatao
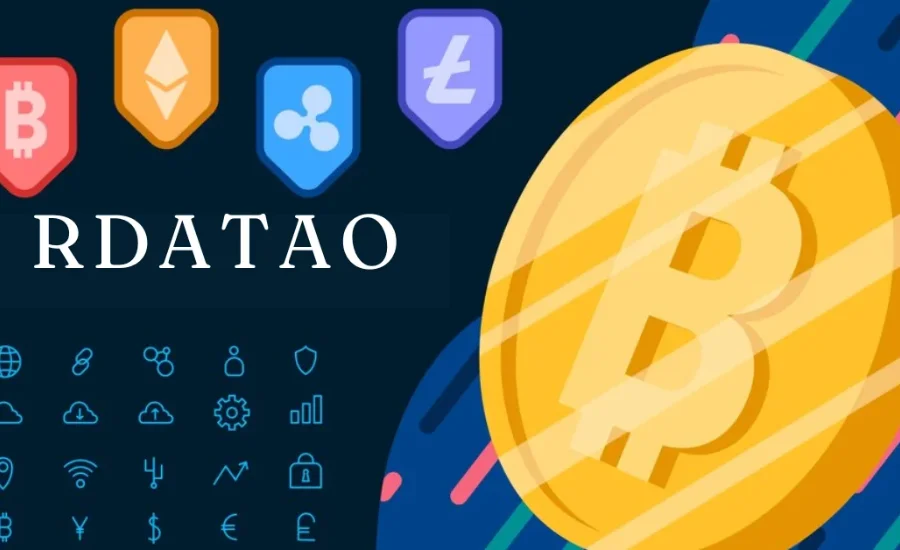
Rdatao follows a structured process to give meaning to raw data. Here’s how it works:
Data: It starts with data collection from databases, sensors as well those available on different online platforms. This data comes in the form of structured formats such as spreadsheets and unstructured forms like social media posts.
Data Cleaning: Then rdatao clean the data by removing any errors, duplicates, and inconsistency in order to make sure that its accurate and reliable.
Data Transformation: The processed data is transformed to setup the analysis. This step would include scaling units, classifying data and linking the details to make sense of it all.
Data Storage: Written data is stored in a database or data warehouse for future access.
Data Analysis: Advanced methods and machine learning algorithms are used along with statistical methodologies for rigorous analysis of the data, in order to pull out hidden insights.
Results of Analysis Displayed through visualizations, charts and graphs. This visualization makes understanding easy and also helps in Decision-Making.
Through these steps, rdatao helps organizations efficiently to navigate and derive value from massive and complicated datasets. This way it uses specialized software and tools specifically designed to handle large-scale data operations enabling users in identifying trends, predicting outcomes and supporting the dissemination of well informed decisions.
Selecting Tools: Upskilling Data Inspection
When it comes to the data analysis world, your choice of tools and software can have a profound influence over how you go about doing things – or even what insights come from them. There are so many options available and each of them cater to different needs; hence taking an informed decision is very important.
RDatao is one of the most widely used tools by data analysts for statistical computing and graphical representation. The drilldown of the GUI makes it easier for users to create complex analyses in an efficient manner.
Python, an advanced programming language also provides several libraries like Pandas and NumPy to do hard tasks of data manipulations as well as for various types of visualisation. For more specialized functionalities, tools such as Tableau or Power BI can create dynamic dashboards and full-fledged reports.
The Connection between Data Quality, Prep and Being a RuggedData Scientist
The groundwork for a successful data analysis is the health and preparation of your datasets. Accuracy, Completeness and Relevance: To derive the proper insights which are relevant to our exercises we need it to be accurate & complete. Results can be skewed and decisions lead astray by inferior data.
Data preparation involves cleaning, transforming and structuring data to boost its analysis as a whole. This involves adding missing records, removing duplicates, and bestowing the uniformity of formats to ensure consistency.
Kind of like that hard staking for 2 hours, only less painful and instead you can feel quite smug about because it will benefit the longevity of your data project. By having a proactive approach like this, you are able to catch potential pitfalls downstream.
So, the saying “garbage in garbage out”, no matter what analytical techniques or tools you apply to bad data it will yield poor results. Making data integrity a priority is about the credibility and usability of your analytics efforts over time.
It is defined by the tool which most closely meets your needs and skill set. However, by stepping through a variety of options you can quickly figure out what the best way is to fulfil requirements for data analysis.
Navigating the Data Analysis Journey: Key Steps for Success
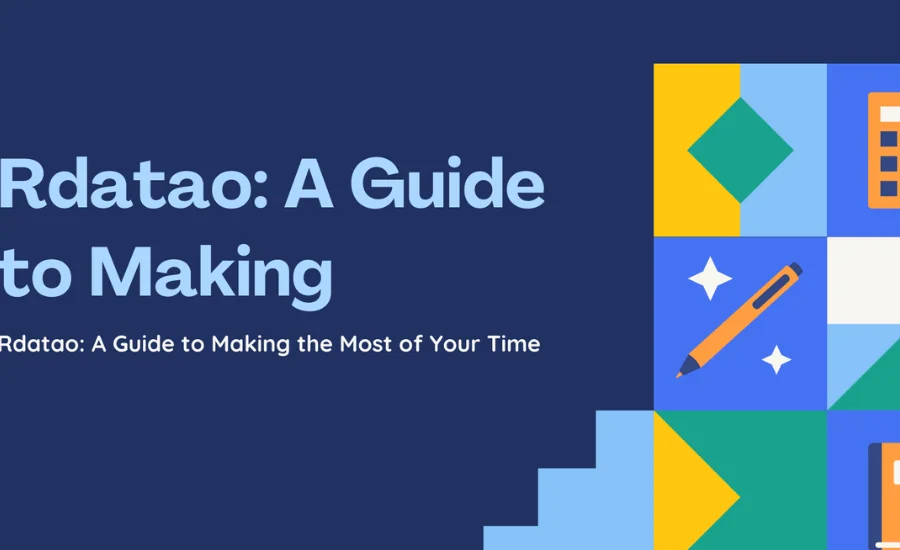
Data analysis unfolds as a methodical journey encompassing inspection, refinement, transformation, and modeling of data to unveil insightful discoveries. It commences with defining the core problem or inquiry that serves as the focal point guiding the entire process.
Once the objective is established, the subsequent step involves gathering pertinent data from diverse sources, prioritizing accuracy and comprehensiveness for robust analysis.
With the data assembled, the preprocessing phase ensues, focusing on cleansing and structuring the data for optimal usability. Tasks here include rectifying missing entries, eliminating anomalies, and standardizing variables to ensure uniformity.
The analytical journey then advances into exploring the data using descriptive statistics and visual aids such as histograms and scatter plots. These tools illuminate underlying patterns and correlations embedded within the dataset.
Subsequently, hypothesis testing and modeling enter the stage, employing statistical methodologies to derive insights or forecast outcomes based on earlier data revelations. Effectively interpreting outcomes and articulating discoveries with clarity mark the culmination of a successful data analysis endeavor.
Navigating Challenges in Data Analysis: Strategies for Success
Data analysis often presents a spectrum of obstacles, from managing incomplete datasets to selecting optimal analytical methodologies. A fundamental hurdle involves ensuring data integrity and precision before embarking on analysis. Addressing this entails rigorous data cleansing procedures and meticulous verification of dataset validity.
Another critical challenge lies in choosing suitable tools and software amidst a plethora of options available. Selecting tools that align closely with specific needs and expertise is paramount. Investing time in mastering these tools enhances analytical prowess significantly.
Furthermore, accurately interpreting results and deriving meaningful insights from intricate datasets can prove daunting. Overcoming this challenge necessitates continuous refinement of analytical skills through practice and staying abreast of evolving data analysis trends.
Collaboration with peers and seeking guidance from seasoned professionals can also yield invaluable perspectives, facilitating more effective resolution of complex data analysis challenges.
Impact in the Real-World on Data Analysis In Different Sectors
There are tonnes of real-world examples in different sectors that show just how useful data analysis can be. For instance, data analysis is crucial in healthcare for predicting patient outcomes and perfecting treatment protocols to help deliver better medical care.
Data is a very important anchor for the more tactical side of marketing because with data you can be strategic i.e. – manufacture or marry products and services to consumer behavior using advertising, significantly lowering your cost per acquisition (CPA) while gaining monetizable hints at how best to engage new customers within specific margins only loss-tolerant mar-tech companies would approach successfully.
Banks use data analytics to detect fraud in real time and get comprehensive market intelligence on which to base their investment decisions.
The same is happening in transportation, where analysis of traffic flow helps to better route planning and reduces congestion, thus increasing urban mobility.
Data analysis is the cornerstone of e-commerce, helping companies to understand their customer likes and dislikes so that they can recommended products which sell well – in turn boosting both sales and increasing consumer satisfaction overall.
In education, data-driven insights help educators keep an accurate record of student performance metrics to adapt personal learning algorithms aimed at meeting the unique needs.
This variety of uses demonstrates how data analytics can drive innovation, ROI and strategic decision-making across industries.
The essential of rdatao
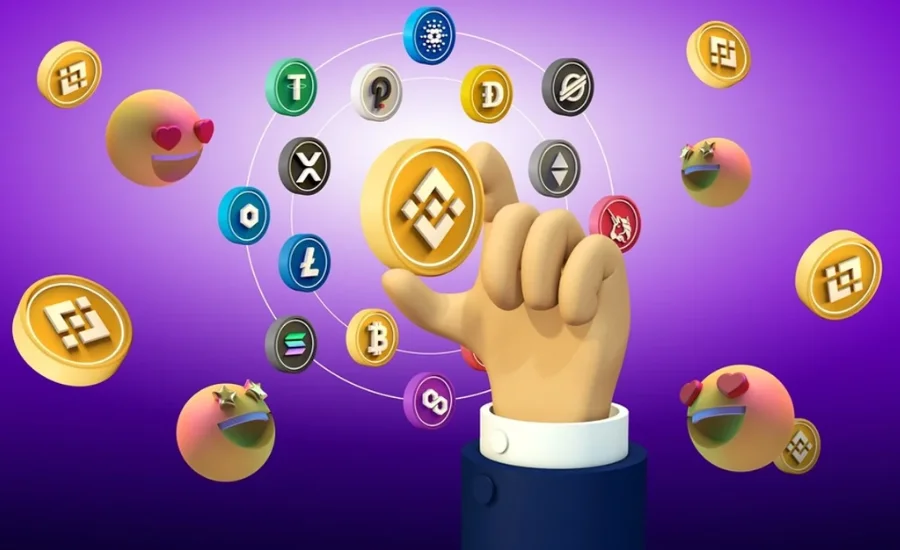
Rdatao provides a number of benefits for improved decision making and operational efficiency Rdatao is used across the sector in various ways like :
Better Decision Making: rdatao provides insights by analyzing huge datasets which lead to the better decision making aspect. This means businesses use rdatao to Identify customer preferences, pinpoint inventory management & develop intelligent marketing strategies. Healthcare: Health is another one of those fields, rdatao helps in proper disease diagnosis and planning effective treatment methods tailor-made to every patient for better medical results.
Efficiency: rdatao automatically processes your data to save processing time and eliminate many common errors associated with manual handling. This efficiency enables organizations to better allocate human resources so that they can focus on more value-added tasks, and lessen the burden from routine data work.
Accuracy: Advanced algorithms of rdatao which are built-in it make better data analysis than human skilled. They can be trained to detect elusive patterns in big data which are much harder for a human – even one with years of experience as an analyst -to pick up, making the AI both enable more accurate predictions and inform context-aware decision-making.
Most Large Scale data: Rdatao shines the best when handling large scale data operations, which is why it perfectly suits for projects regardless of being small or big ones. With rdatao, enterprises can easily analyze even hundreds or thousands of datapoint/records in a go while keeping the performance level as they desire.
With these qualities rdatao has become essential toolkit for any organization aiming to fully leverage what their data provides. Around Factor AnalysisWith rdatao, companies can analyze more deeply to optimize processes and gain competitiveness in their markets.
Tomorrow is Coming: How Data Analysis has Developed
Breaking Barriers: The Future of Data Analysis as Technology Steps Forth Now, data analysts are on the edge of an enterprise transformation driven by artificial intelligence and machine learning that will allow them to unearth complex patterns and trends in huge datasets faster than ever before.
Businesses will look for the capability to gain actionable insights on aspects such as real-time analytics rapidly. These will enable organizations to become more agile in their analytical processes, thereby allowing them not only react faster but also respond with data- driven decisions.
Data Privacy will emerge as major consideration and having adherence to standards of gdpr will play a crucial role. It will be important to maintain data practices that are transparent, accountable in order to have trust among consumers and stakeholders.
Over the horizon, more possibilities flourish in technologies such as predictive analytics, augmented analytics and automated decision-making systems. In the years to come, these technologies are poised to change how organizations can extract insights from data in order for them innovate faster and operate better.
Challenges in Implementing rdatao
rdatao offers major benefits, but it also poses organizational challenges that need some discussion:
Complexity – rdatao systems are not for the faint of heart, requiring expertise in data science/programming/domain basics. Training or employing the right people is often required to effectively design and maintain these systems so that they may work best for your business.
Cost: Getting rdatao systems setup and working can be a significant cost. And then you will have either monthly fees for someone to maintain the system or in-house personnel that may have additional expenses such as health insurance, by itself. That includes purchasing the hardware, software and hiring professionals to work on it. More often than not, however, the heartbreak of losing time and throwing good money after bad—resources that could have been invested elsewhere as an alternative to rdatao—more than justifies those investments in both efficiencies and knowledge over time.
Data Privacy- Handling huge data creates privacy and security issues. Companies must comply with strict data protection laws, and take all possible security measures to protect sensitive information. This can hurt you, when the data breaches occur financially and more importantly tarnish your reputation.
Reliability: As with any analytics system, the trustworthiness of insights produced by rdatao will be determined by the quality of data behind it. Data that is low-quality, inaccurate or inconsistent can affect the results of data analysis and ultimately calls into question decisions. Data quality caring requires super-protective data cleaning and validation process, as well continuous alertness to detect special cause or common causes of variation.
Despite these complexities, when solved effectively this work allows an organization to tap into the transformational power of data. These obstacles can be surmounted by businesses that wish to unchain insights and drive efficiencies in the already fast-paced, data-centric world different entities compete against each other.
Analyzing the Advantages and Challenges of rdatao
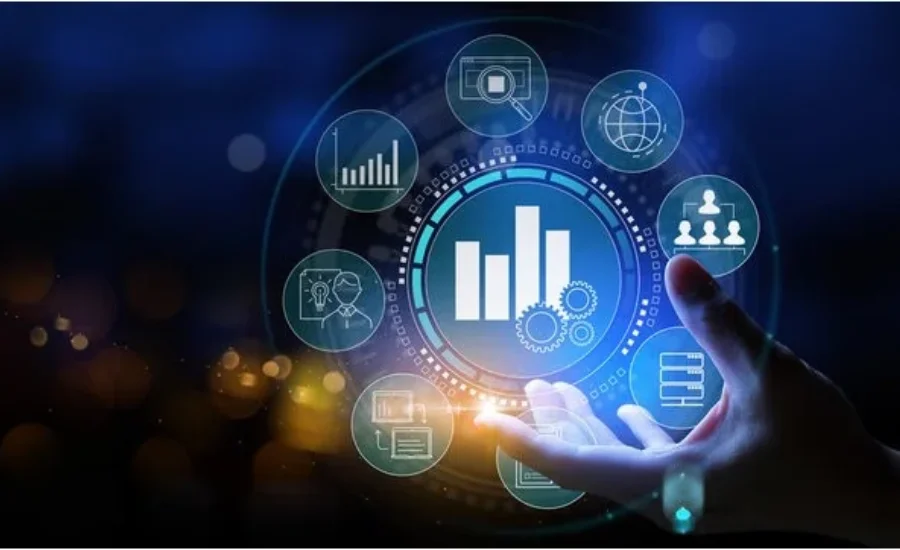
Pros
- Improved Decision Making: Rdatao enables swift and accurate analysis of large datasets, empowering organizations to make informed decisions based on data-driven insights. For instance, businesses can optimize inventory levels by analyzing sales data, leading to enhanced resource allocation and profitability.
- Efficiency: Automating data processing tasks through rdatao reduces manual effort and time required for data management. This efficiency allows teams to focus on strategic initiatives while accelerating routine processes like data cleaning and transformation.
- Accuracy: Leveraging advanced algorithms, rdatao minimizes errors inherent in manual data handling. Automated analysis ensures consistency and reliability in insights, bolstering decision-making processes across various domains.
- Scalability: Rdatao systems seamlessly scale to accommodate large volumes of data, maintaining performance integrity. This scalability is critical for organizations dealing with substantial datasets from diverse sources, facilitating comprehensive analysis and integration.
Cons
- Complexity: Implementing and maintaining rdatao systems demands specialized expertise in data science and IT infrastructure. Organizations may need to invest in training or hire skilled personnel, posing challenges, particularly for smaller entities with limited resources.
- Cost: The initial setup and ongoing maintenance of rdatao systems entail significant financial investment, encompassing hardware procurement, software licenses, and personnel costs. While the long-term benefits justify these expenses, upfront costs can be prohibitive for some organizations.
- Data Privacy: Managing extensive data raises concerns regarding privacy and security. Organizations must uphold stringent data protection measures to safeguard sensitive information and comply with regulatory requirements. Ensuring robust security protocols and maintaining compliance can be resource-intensive and complex.
- Data Quality: The efficacy of rdatao hinges on the quality of input data. Poor-quality data can distort analysis outcomes and undermine decision-making accuracy. Maintaining data integrity necessitates rigorous validation, cleaning, and ongoing monitoring efforts to derive dependable insights.
Navigating these pros and cons effectively allows organizations to harness the transformative potential of rdatao while mitigating challenges. By addressing complexities through expertise, managing costs judiciously, prioritizing data privacy, and upholding data quality standards, businesses can leverage rdatao to drive innovation, efficiency, and competitive advantage in their operations.
Final Words
Harnessing the power of data analysis through tools like rdatao can revolutionize how organizations operate. By enabling accurate insights, improving decision-making, and enhancing operational efficiency, rdatao empowers businesses to stay competitive in today’s data-driven world. Embracing these technologies with a focus on data quality, privacy, and scalability ensures that organizations can maximize the benefits while navigating challenges effectively.
FAQs
1. What is rdatao?
- Rdatao is a comprehensive data analysis tool that automates the processing, analysis, and visualization of large datasets using advanced algorithms and statistical methods.
2. How can rdatao benefit organizations?
- Rdatao improves decision-making by providing accurate insights from data, enhances operational efficiency through automation, and scales to handle large volumes of data effectively.
3. What are the challenges of implementing rdatao?
- Implementing rdatao can be complex and costly, requiring specialized expertise and significant initial investment in hardware, software, and personnel. Organizations also need to address data privacy concerns and ensure data quality for reliable results.
4. How does rdatao ensure data privacy and security?
- Rdatao systems implement robust security measures to protect sensitive information, complying with data protection regulations and ensuring secure data handling practices.
5. What steps can organizations take to maximize the benefits of rdatao?
- Organizations should invest in training for personnel, prioritize data quality maintenance, and continuously update their systems to leverage new advancements in data analysis technology.
To stay updated with every kind of news folloe us and join us on Stellar Whirl